Mapping and modelling made easier with AI
|
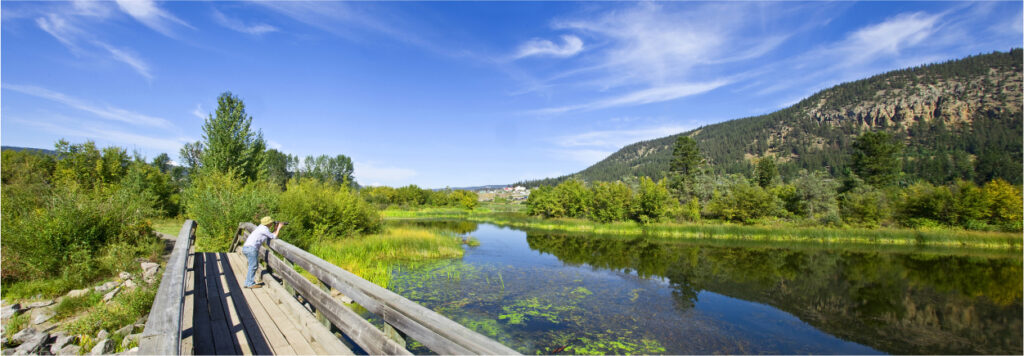
At the Ministry of Water, Land and Resource Stewardship (WLRS), mapping is used for managing natural resources, ecosystems, water, biodiversity, forestry and food production.
Mapping ecologically diverse areas and interpreting the results are tough to do, even with a trained eye. Identifying features like wetlands can depend on details like the structure of the underlying soil and fluctuating water levels which are not easily observed using topographical data and aerial photography. This often results in some wetland areas being overlooked and not included in the mapping, which leads to operational issues where development plans overlap with existing wetland areas.
To improve overall mapping and data, GeoBC’s Ecosystem Information Services (EIS) team is using machine learning (a form of AI) to map landscape characteristics and support better resource planning and decision-making. These innovative tools have enabled filling spatial data gaps in a cost-effective way, building repeatable transparent processes, and creating consistent, scientifically defensible mapping.
The status quo
The team at EIS are working on a variety of predictive mapping projects with a wide range of partners and funders, from predictive ecosystem mapping with the Ministry of Forests to advanced digital soil mapping with SFU, Dalhousie, UBC and other academic institutions. Field data for these projects were gathered in partnership and collaboration with several First Nations, including McLeod Lake, Prophet River, Tsay key Dene and Taku River Tlingit, with the shared goal of improving forestry, water stewardship and climate science.
But the status quo includes some challenges. B.C. doesn’t have province-wide coverage for the finer details of landforms and what may be under the surface. There are gaps in the raw data and mapping that describe geological features which make it difficult to conduct provincial, regional, and even localized analyses. In addition, there is no single centralized information system or structure, so data is stored across multiple agencies and databases in a variety of formats, leading to a lack of harmonization and structure.
With these limitations in mind, EIS established a pair of objectives to ensure better data, and in turn, better mapping:
- Develop a framework and standardized system, creating uniform categorization which supports various types of mapping (groundwater, etc.) for the future and can enhance our understanding of the landscape
- Develop a workflow to map the landforms, further enhancing the robustness of the ecosystem mapping process
Sorting the data
Machine learning models are only as good as the data upon which they are built. Good data is needed to train and validate any predictive mapping produced with AI. This requires statistically valid sampling, clean and organized data and an in-depth understanding of data limitations.
Mapping data for different types of land includes mountains, plateaus, and valleys/lowlands, each with their own characteristics of bedrock, slope, sediment and soil.
EIS harmonized their classifications by combining compatible categories of soils, surface geography and terrain mapping, to apply a machine learning approach for modelling and creating predictive maps.
Feeding the machine
In mapping and modelling, AI is used widely; however, the field lacks standards and best practices around appropriate use of data for training and validation. Additionally, methods of collecting new data for machine learning differ from traditional sampling and inventory practices.
To set their machine learning models up for success, the team at EIS created multiple sets of training points for their region and modelled different combinations, tracking terrain attributes like elevation, slope, ruggedness and ridge/valley flatness.
The team started with 157 of these attributes and optimized their settings for each run of the machine learning model, testing and retesting multiple classes to detect broad landforms, landform subclasses, aquifer classes, surface expressions and surficial materials.
To help classify their results, the team used an algorithmic model called “Random Forest.” This is a commercially available product that doesn’t require a supercomputer to run and can easily be used to examine ecological environmental data, even by people who aren’t technical experts.
In mountainous areas with a thin till veneer over rock and thick glacial deposits in the valley, the machine learning model detected lower slope deposits that Quaternary geology mapping had missed, impressing the team.
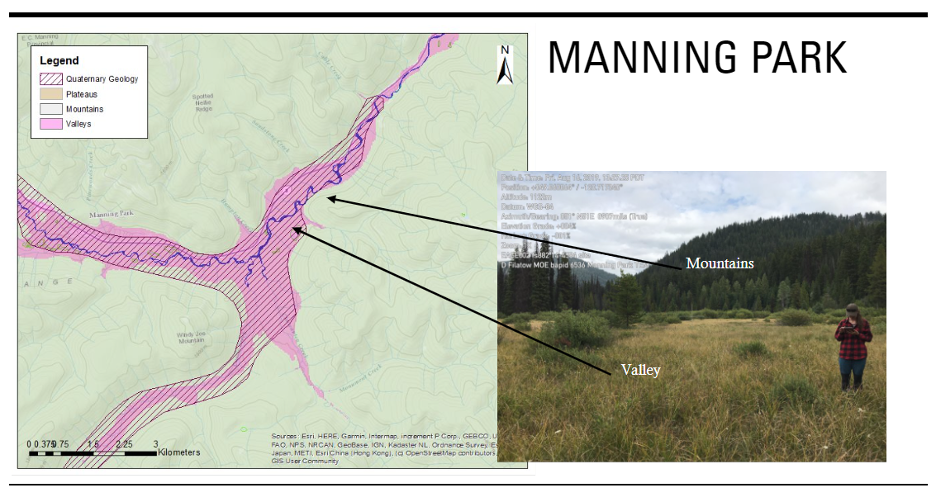
Mapping what’s next
Maps produced through AI provide cost effective alternatives to traditional mapping and can improve our understanding of British Columbia’s diverse landscape. Using these maps to understand spatial patterns and relationships will lead to better informed land management decisions for a resilient future. Improvements in computing capacity, field data, expertise, and improved coverage and resolution of input layers (such as LIDAR) will bring better accuracy and help to further expand this work.
By making their data and scripts available under open licensing, EIS aims to facilitate sharing and growth of machine learning in mapping and modelling landscapes in B.C. The team hopes to continue making progress in these areas, and to garner support to extend their mapping across the province.